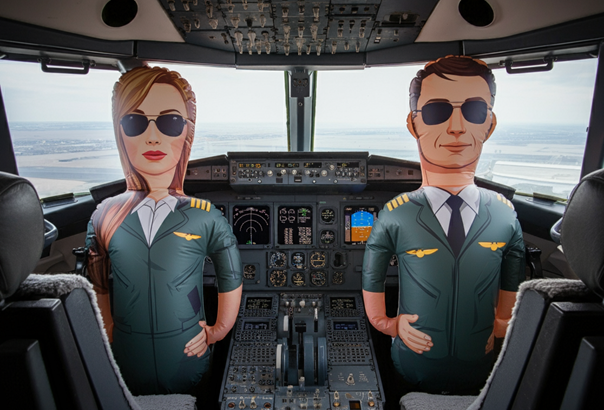
Cutting through the AI fog
With so much hype surrounding generative AI (Gen AI), and so many people promoting AI co-pilots as a way to boost productivity, is there evidence to suggest that “contract co-pilots” are ready for prime time? How do you decide when to use these tools, and what guardrails are required to ensure they don’t create more problems than they solve? The best answer seems to be that while Gen AI is pretty good at analyzing contracts, it cannot yet be trusted to do so reliably (at least, not on its own). You either need to build expert human guardrails or find tools that have robust built-in features for sorting good data from bad. Blindly trusting a generic Gen AI co-pilot will probably send you skidding off the runway.
Some of what Gen AI can do is unequivocally impressive. The chat-bot experience is natural, flexible and often fun. People love chatting, especially to a conversationalist with seemingly endless encyclopedic knowledge. So, it’s no surprise that many people are enthusiastic about the potential of this technology.[1]
For contracts, reliability matters
But, however fun it is to play with ChatGPT,[2] research shows that reliability is a problem, for a couple of reasons.[3]
First, the very nature of Large Language Models (LLM) chat-bots[4] is that they are stochastic (randomly determining) guessing machines, doing their utmost to give you a response that sounds real and plausible when you tell or ask it something. This means they can give a different answer every time you ask them the same question. (Or you might often get the same answer, but you never know when you’re going to get a different one.)
So, keep in mind – variability might be fine for creative use cases where there is no right answer, like if you need a joke to break the ice when presenting to a room full of contracting professionals. But it’s not okay to get randomly different answers to questions with high levels of business and legal risk. If a chatbot skips over the most favored nation pricing clause when summarizing a contract, that could turn into a very expensive mistake.
Second, and perhaps more dangerous, is the tendency of most LLMs to make things up. In response to certain questions, generative AI chatbots will sometimes give answers that are completely untrue. Industry jargon for these factual errors is “hallucinations,” although, unlike human hallucinations, which are inherently nonsensical, AI hallucinations are designed to look and feel deceptively real. For contracts, this a significant problem. A hallucinating AI might provide data suggesting that Acme Ventures LLC is your contractual counterparty, when in fact that party is Ace Ventures LLP. For reasons that remain mysterious, an LLM might just decide that Acme Ventures LLC sounds like a more plausible answer. Reporting false counterparty data like this to a regulator would almost certainly be a serious compliance failure.
Hallucination is real
Which begs the question, how bad is the hallucination problem? Is it so bad that you should avoid AI chatbots completely until further notice? Or is it so rare that you can treat it just like any other data quality problem, and accept a small risk of error? The answer seems to fall somewhere in the middle. Independent academic research suggests that some of the big names of legal and professional publishing offer generative AI products that hallucinate as often as 20-30% of the time.[5] Sometimes it will be less than this, sometimes more, but the 10-20% ballpark is probably a fair generalization right now.
True, hallucination rates can be lowered using a technique called retrieval augmented generation (RAG),6 but this does not eliminate the problem. RAG simply steers a chatbot toward a more factually grounded answer, it does not guarantee a factually reliable answer. In our own internal research at Catylex we have used RAG to improve Gen AI accuracy, for example, by asking the AI to focus only on short, relevant sections of a contract. But even in this scenario we see examples of hallucinations, including fabricated party names, fabricated effective dates, and fabricated data inside financial tables.
To give a real-world example from Catylex internal research, a sample page image from a contract was presented to an LLM with prompting to extract the party names. In this particular example, the executed contract contained a party name with a spelling mistake, namely “Jaris Exhibiton LED Video Services, LLC” (note that an “i” is missing from the word “Exhibition”). Sometimes the LLM quietly corrected the spelling, and therefore inaccurately reported the party name as it was expressed in the contract. Sometimes the LLM preserved the spelling error, accurately reporting the name as written in the contract. While it might be useful to avoid spelling and grammatical errors when generating new content, it is much less desirable for analytical tasks, especially when it might obfuscate contractual errors and risks.
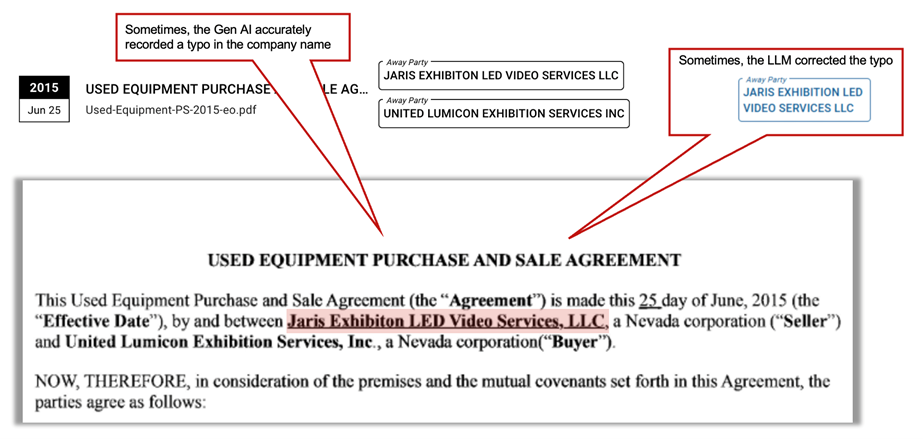
Another example illustrates the risk of glossing over important legal nuances. When asked to provide the contextual language of a limitation of liability clause “without modifying the wording”, the LLM went right ahead and modified the wording anyway. The actual clause said this:
Company shall not be responsible or liable to Client for any loss or damage that may be occasioned by or through the acts of negligence or omissions of any party occupying the Premises…
The LLM hallucinated misleading changes, with this response:
Company shall not be responsible or liable to Client for any loss or damage that may be occasioned by or through the acts of omissions or commissions of any party occupying the Premises…
This changes the meaning of the provision by saying that it applies to all positive acts of an occupying party, rather than just the negligent acts of such a party. This major change of meaning was completely made up (hallucinated) by the LLM.
If we wait long enough, will the big tech vendors eventually eliminate hallucinations? Maybe, but they have not done so yet, and Google’s CEO has described hallucinations as an inherent feature, not a bug.7
Humans to the rescue
Some will argue that contract co-pilots can get away with a few reliability problems, because they will always be teamed up with an expert human. This is probably true for low volume use cases, like reviewing new deals on counterparty paper, where the expert has time to check everything and clean up any mistakes. But it does require significant expertise and vigilance on the part of the reviewer. When some technologies fail, they do so in clear and obvious ways. Optical Character Recognition (OCR)8 errors, for example, typically manifest as a garbled sequence of nonsense characters. Not so with generative AI. When LLM chatbots hallucinate answers, they usually have the appearance of validity. Human experts really need to pay very careful attention to spot them.
Autopilots scale where co-pilots don’t
For large scale contract review, an unreliable co-pilot is a bigger problem. It’s one thing to have an expert checking a dozen contracts every day. It’s quite another to be checking 12,000 or 120,000 contracts in order to weed out AI mistakes. What you really need at scale is an autopilot, not a flaky co-pilot. To get insight across a large contract portfolio (which is the nirvana most contracts professionals dream about), you need the AI to operate autonomously, quickly and cost-effectively to end up with data you can trust, at least for some large, specific part of that portfolio. This has been a major frustration for anyone using AI for large contract reviews. The AI may be correct 80% or even 90% of the time, but there is no way to tell which 80% is correct. To get trusted high-quality data, your experts need to check everything.
Does this mean the dream of portfolio-wide insight is dead for all but the most cashed up projects? Not quite. Trust-worthy autopilots are starting to emerge. For example, some vendors are developing techniques for applying multiple layers of AI to deliver data at scale and to isolate the data that’s most likely to require human validation. By combining layers of deterministic AI (hallucination-free) with generative AI (hallucination-prone) they are able to produce very high-quality data for a well-defined set of contract metadata and documents.
What should contract professionals be doing right now?
So, what practical actions could you, as a contracts professional, be taking right now to leverage AI and solve important business challenges? On the one hand, nobody wants to be the victim of hype or to champion another technology failure.9 On the other hand, many executive teams are looking very seriously and favorably at AI right now, especially where the technology can deliver measurable business value. You do not want to be sitting on the sidelines when your competitors are using AI to achieve a market advantage.
One clear contender for AI action is to carry out data and risk analysis across a large portfolio of contracts. This was the most highly ranked use case in a recent World Commerce & Contracting (WorldCC) AI survey, and it also aligns with how most of your peers are evaluating AI today.10 For good reason. Having machines analyze contracts at scale allows you to discover and eliminate serious risks that would otherwise not be discovered until too late. If AI can successfully automate the analysis task, the costs are dramatically lower than human contract reviews, and the return on investment (ROI) is very compelling.
Here are some practical tips for tackling portfolio risk analysis successfully:
- First, identify some AI-powered products that claim to extract high-quality data autonomously, without significant human effort. Check references with real customers to verify these claims. A shortlist of two products will help to illustrate strengths, weaknesses and similarities, without creating too much overhead.
- Second, identify a selection of your contracts that allows you to test those products and measure their performance. Around 1,000 is a good number, because it will (a) include a diversity of examples, (b) give a sense of how things will scale, (c) not be too much of a burden to evaluate, and (d) call attention to anyone using human reviewers in the background.
- Third, run your tests independently of any vendor involvement and verify how things work with your own eyes. Make sure that data is sorted into clear categories of “auto verified” and “needs checking,” so you know how much of the autonomous extraction you can rely upon with no human effort.
- Finally, if you like the results, scale up. If none of the results are satisfactory, you’ll need to rethink the scope your project in a way that factors in the time and cost of human quality control.
About the Author
Jamie Wodetzki is a legal tech veteran, entrepreneur. He was co-founder and chief product officer of Exari, a global CLM leader acquired by Coupa in 2019. Having spent more than 10,000 hours analyzing and automating technology, finance, insurance, real estate, healthcare, procurement, sales, licensing and other contracts, Jamie has unique insights into contract management. Prior to joining Catylex, he spent 2 years as VP of Contract Strategy at Coupa and 19 years at Exari. Jamie’s other achievements and roles include co-inventor of various contract-related patents; Fellow of the Center for Legal Innovation at VT Law School; Senior Associate at the Minter Ellison law firm.
About Catylex
Catylex is a contract data platform that uses ensemble, multi-model AI to translate legal language into useful data, providing out-of-the-box answers to businesses that depend on contracts. Catylex scales to tens of thousands of agreements, delivers insight and quality superior to any single LLM and supercharges contract review—helping legal, risk, compliance, procurement, and C-Suite teams unlock the value hidden in their agreements. Learn more at catylex.com.
END NOTES
- Survey data shows that personal enthusiasm for AI increased substantially from 2023 to 2024, more than doubling from 36% to 76% - see “AI in contracting: untapped revolution to emerging evolution”, World Commerce & Contracting, February 2024, https://www.worldcc.com/Portals/IACCM/Reports/AI-in-Contracting.pdf.
- ChatGPT is a large language model, a type of generative artificial intelligence, developed by OpenAI. It's designed to understand and generate human-like text, allowing for conversational interactions and various text-based tasks. (AI Overview definition).
- Princeton academics Narayanan & Kapoor explain the importance of reliability in this post (https://www.aisnakeoil.com/p/ai-companies-are-pivoting-from-creating), based on their recent book, AI Snake Oil: What Artificial Intelligence Can Do, What It Can’t, and How to Tell the Difference, September 2023, Princeton University Press.
- LLM chatbots leverage Large Language Models (LLMs) to create more natural and contextually relevant conversational experiences, going beyond traditional chatbots by understanding and responding to complex queries with greater adaptability. (LLMs employ machine learning models that use mathematical calculations based on an input to produce an output. (CNET article.)
- “Hallucination-Free? Assessing the Reliability of Leading AI Legal Research Tools”, Magesh and others, Human-Centered AI group, Stanford University, https://doi.org/10.48550/arXiv.2405.20362. See also “Large Legal Fictions: Profiling Legal Hallucinations in Large Language Models”, Journal of Legal Analysis, Volume 16, Issue 1, June 2024, https://doi.org/10.1093/jla/laae003.
- Retrieval-Augmented Generation (RAG) is a method that enhances the capabilities of Large Language Models (LLMs) by combining them with external knowledge sources. It works by first retrieving relevant information from a knowledge base based on a user's prompt and then using this retrieved information to augment the LLM's generation process, leading to more accurate and contextually relevant responses. (AI Overview). See also Amazon Web Services article.
- “Google CEO Sundar Pichai on AI-powered search and the future of the web”, interview with Nilay Patel, Editor in Chief of The Verge, https://www.theverge.com/24158374/google-ceo-sundar-pichai-ai-search-gemini-future-of-the-internet-web-openai-decoder-interview
- OCR is Optical Character Recognition, a technology that converts scanned images or digital photos of text into machine-readable, editable text. This allows users to manipulate the text as if it were typed, including searching, copying, and editing it. (AI Overview)
- A recent Rand Corporation research paper reports that AI projects have an 80% failure rate: “The Root Causes of Failure for Artificial Intelligence Projects and How They Can Succeed”, August 2024, https://www.rand.org/pubs/research_reports/RRA2680-1.html
- WCC, AI in contracting, February 2024, ibid.