Originally published June 30, 2022, this article is reprinted by permission from Andrew Moorhouse, Director of Consulting, ALITICAL Ltd.
Predicting NPS is ‘the’ burgeoning topic in enterprise contact centre analytics. But predicting NPS is hard; damn hard. And are the tech vendors getting it right? Or just cobbling together a predictive ‘AI’ solution, based on trite agent behaviours?
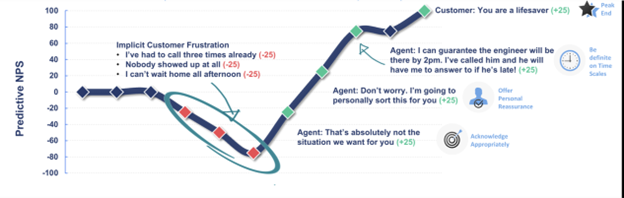
Image courtesy of ALITICAL Ltd. A model for predicting NPS1
This unashamedly long-read shares conversational intelligence from 15 years' worth of enterprise call listening projects and suggests elements you may wish to consider if building your own in-house predictive NPS measures. This isn’t a thinly-veiled whitepaper, don’t worry.
Neither is it a formal Gartner-style assessment, but I will prod and poke the various vendor offerings and share my thinking. I’d suggest that despite the promises of ‘out of the box’ vendor functionality, your business is unique. And requires a unique approach for accurate NPS predictions. Generic approaches beget generic results.
Using 10,000 LivePerson Live Chat Transcripts, we were able to model 91% of the variability in NPS scores. This article is an impassioned rant as always.
Why we must take a step back and think
“Just tell them to add more,” boomed the air-force commander. “We need more armour on the wings and tail.” It was 1943, and inside a giant hangar at the Center for Naval Analyses, over 40 airforce and naval personnel were inspecting bullet-riddled planes that had returned from WWII sorties. “Look at all of these bullet holes, the wings are riddled. That's where we need more plating.” The room full of engineers, officers and senior airforce personnel all nodded in unison. All except one.
A slim, bespectacled professor cleared his throat and stepped forwards, “Ahem.” he stuttered, “What statistical assumptions exactly are you making here?” The alpha-male crowd disparagingly turned to face him. “You see,” the professor continued, “My analysis* suggests you are making a logical error by looking only at planes that survived; what you are missing are the planes that didn’t make it back. We don't need more armour on the wings or tail. You need to put the armour where there are no bullet holes.”
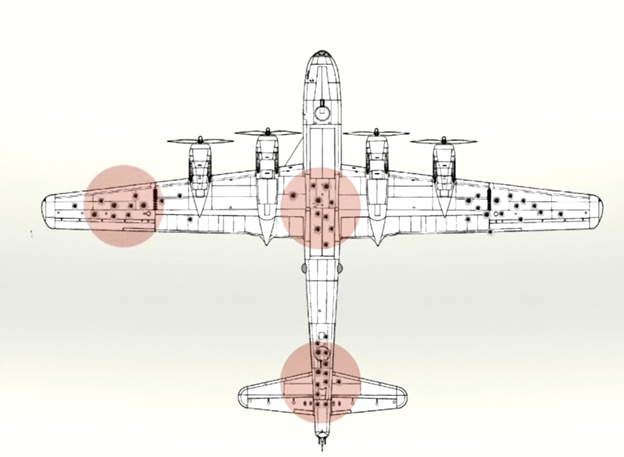
It was an a-ha moment. A rare occasion where logic was persuasive and changed the current thinking. Professor Wald surmised that the engines were the most vulnerable: if they were hit, the plane didn’t return to be counted in the research. The military listened and armoured the engines, saving tens of thousands of allied lives. Professor Wald sadly died in 1950 but his legacy and theory of survivorship bias live on.
Survivorship bias describes the logical error of looking only at subjects who’ve reached a certain point without considering the (often invisible) subjects that never made it.
Cute story…right? Yes. But what’s the relevance to your contact centre and predicting NPS? You see, to predict NPS you need to consider the interactions that didn’t make it. The plane crash conversations that never survived. Just one Luftwaffe 20-caliber bullet (or corrosive agent behaviour) may ensure the entire interaction crashes in the NPS Detractor / CSat1 region.
- *Wald’s findings were far more nuanced than this. And utterly fascinating. His work was declassified in 1980 and the full 100-page reprint is listed in the Sources section at the end, should you want a geek-out moment!
Predicting Plane Crash Conversations (aka NPS Detractors)
The logical Wald-esque error being made today in predicting NPS from customer voice and (more rarely) chat interactions, is most approaches exclusively look at the agent utterances believed to improve CX. The "smile when you dial" behaviours.
But no matter how much armour (aka canned empathy) is added, certain conversations do not survive. Let’s dive into the horrific trend of canned empathy; then look at the other myths around predicting NPS. The article will then share what we found when attempting to predict NPS.
Canned Empathy is rife (and not sufficient)
There’s an unhealthy obsession with forcing and measuring empathy, to the detriment of the agent behaviours that improve NPS.
Have a look at this canned empathy example. You can tell it’s a copy-and-paste item. There’s no customisation and it’s just perfectly "textbook.”
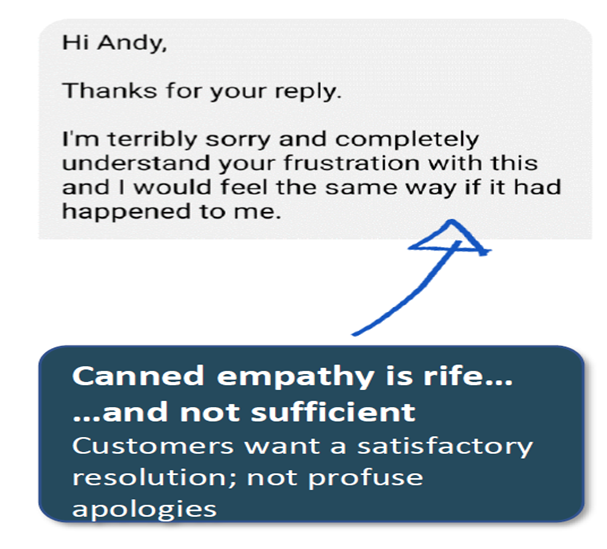
“I’m terribly sorry and completely understand your frustration with this and I would feel the same way if it had happened to me.”
It’s just too fake. Too perfect. Too canned. Ironically, I know definitively this is canned empathy as I worked with the team responsible for upskilling 1,400 agents in delivering empathy. Whoops!
Before going further, whilst I know you know this, I must define empathy for the purposes of clarity. According to the Chief Empathy Officer at Genesys, “Empathy is the ability to listen and understand people’s unique situations.” Fair enough. So, let’s look at how much empathy might be needed in a contact centre.
Fools’ Armour
Let’s take 100,000 inbound calls at a UK High-Street bank. How frequently is empathy required? 100% of all calls? 90%? Maybe 80%? The data below is absolutely robust and suggests that the number is 39%.
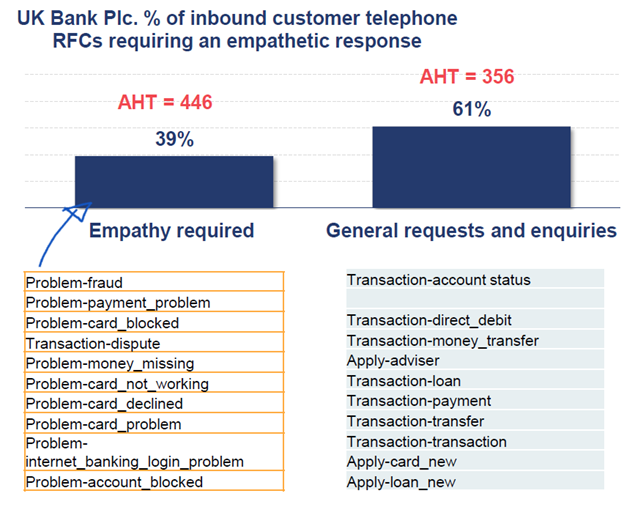
You see, despite the narrative that transactional customer intents have been automated, that’s not the case at all for enterprise contact centres. 61% of inbound traffic in this context is for a transactional request: Cancel a standing order; set up a direct debit; move money.
These are basic telephony transactions. Literally banking transactions. There’s no need for forced empathy and certainly no need for an agent dashboard with a 100% empathy target.
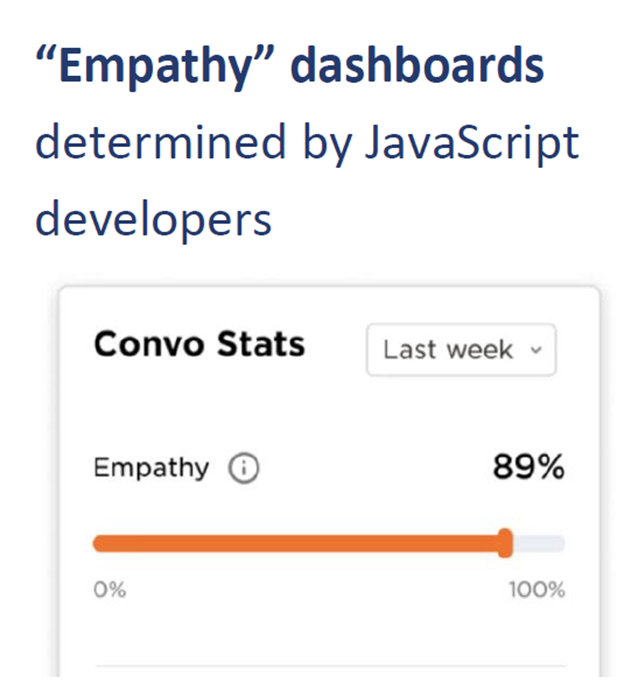
I implore you. Don't implement a 100% Empathy target, when only 39% of your calls require empathy. Yes, it looks sexy and yes, it's measurable. But you are measuring the wrong things. Or at least link it to the presence of customer frustration and/or your customer intents that are identified as requiring empathy.
The drive towards empathy-overload, based on these data, just doesn’t make sense. Nor does adding empathy to a transactional conversation improve NPS.
You can’t bullsh*t a bullsh*tter
Here's the real reason canned empathy will never work. Customers are savvy; smart and easily see-through canned attempts at empathy. Out of all available (public) sources, FaceBook “Social Media Agents” consistently deliver the most cringe-worthy false- empathy. It’s a contact strategy omnishambles and agents often have no “teeth” or system access to actually resolve anything. For one UK socials team, their only go-to-tool is a profuse apology. Here’s what the customers think:
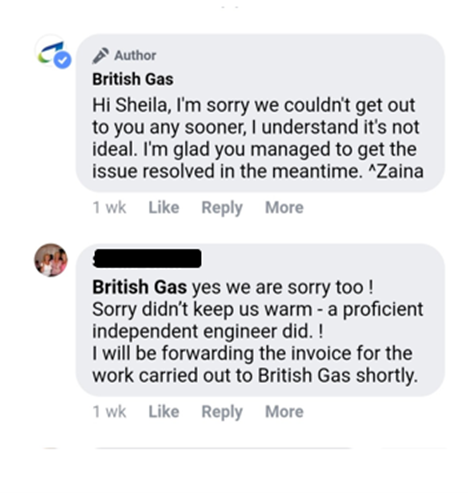
“We are sorry too. Sorry didn’t keep us warm, a proficient independent engineer did”
Empathy is a false god
In the context of predicting NPS, more empathy does not correlate with better NPS. In fact, analysing 10,000 LivePerson Chat Transcripts, there’s a negative correlation. That is, there’s 2x as much empathy in detractor interactions. Of course, we wouldn’t be foolish enough to suggest cause and effect. The high volume of agent placation here is symptomatic of a plane crash conversation, but empathy alone wasn’t enough to fix things, so the interaction crashes in the detractor region.
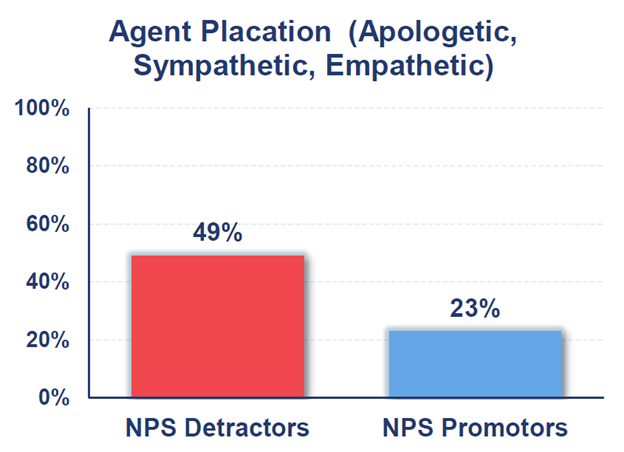
Just because you can measure it… doesn’t mean you should
The Vendor Landscape
Tony Bates, incoming CEO at Genesys appears to have bet the entire farm on measuring and driving empathy, and wants to, “... embed the power of empathy into every experience.” NICE-Nexidia scores your agents out of 10 for being empathetic. Call Miner is less advanced in its thinking. They listen out for a single utterance of the word sorry and claim that “empathy was delivered.” As for Verint, the first time I looked at a large-scale speech analytics installation, Verint had ranked 1,200 agents on how frequently they apologised. Profusely Apologising was deemed to be one of three key employee performance measures. In the sources at the end, I've added a detailed link that shares this story.
There are ZenDesk plug-ins available that rate the agent’s empathy usage out of five-stars on each interaction. But seriously? What does a three-star empathy piece of text look like vs. a four-star version? I’m a conversation scientist and have trained and calibrated thousands of conversations and I don’t believe there’s a sliding scale here. It’s a zero star or one star. It’s a binary assessment. How do you achieve a five-star example without profusely apologising?
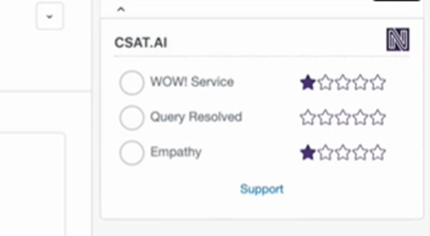
I managed to hit my five-star KPI dashboard by aapologising like crazy. But is this really what customers want? Five trite apologies?
Toxic empathy and the empathy trap
The message is hopefully clear. Empathy alone isn’t sufficient. There’s something fundamentally missing. Empathy without resolution is worthless. Adding more and more empathy to these detractor conversations is like adding armour to the plane wings and tail. The intent is good, but this won’t stop the conversation from crashing into the NPS detractor region.
Of course, you should measure these behaviours, but if you are building a predictive algorithm, the presence of empathy alone will not enable you to predict NPS. Human interactions are far more nuanced than that.
I’d argue customers want easy, low-burden (low effort) resolution. Full ownership by the agent if there is a problem. Ownership to resolution. First-time resolution with no-repeat contact necessary. So, let’s take a little look at ownership and see why this features heavily in the agent dashboards by the big speech vendors.
Delighted and willing to help
Back in 2017, I spent a week with the offshore BPO contact centre team for a global retailer. It was a brilliant operation. Within the team of 1,000 agents, just five years ago, there were only 40 agents on WebChat. Conversational AI wasn’t a thing at that time. There was no app. No asynchronous What’sApp. Just a WebChat portal.
And on a Thursday afternoon, I sat down with the WebChat leader to understand their role, any pre-packaged templates and to glean what I could about what their top performers did differently to drive CSat. The following example is paraphrased, anonymised but the initial conversation opening is verbatim:
Agent: Hi there, I'd be delighted to resolve your query today. I’m Adam. What is it I can help with?
“I’d be delighted to resolve your query…”
Well, my initial reaction was to think, “Daaaamn. This guy is good.” This was a textbook opener. Just brilliant right? I’d be delighted to resolve your query. This is about as good as it gets. Polite, Personable. Willing to own to resolution. All of the best practice agent behaviours rolled into one cute opener. So, let’s progress the conversation:
- Customer: Well, you see, this is the fourth time that I've tried to talk to someone in the customer VIP team. I urgently need to talk with them.
- Agent: Ah, well you've come through to web chat today madam and I can't transfer you.
- Customer: No no no, I understand that's the case. I just need their number. I just need their number because I got cut off earlier so can you just tell me the number?
- Agent Well, no, we're not allowed to give out their number because it's the customer VIP team and a different department. It’s a specialist team.
- Customer: So, so you can't help?
- Agent: No,, you would need to call and get transferred. Is there anything else I can help you with today madam?
The autopsy of a failed live chat
Another example of (human) live chat gone horribly wrong, but that's not the point. Here, the issue, relating to predicting NPS, is the opening statement and the challenges this poses for your predictive AI algorithm.
“I’d be delighted to resolve your query” This is ownership verbalised. Ownership personified and exemplified. But, of course, it’s not ownership at all, is it? Just another canned nicety. A trite, pre-packaged statement.
But run this through any predictive CSat / NPS AI tool and what do you get? Well, you’d get a tick in the “ownership” box for sure. Right? Because it doesn't get better than “I’d be delighted to resolve your query.” So whilst we want ownership. This isn’t ownership. And you won't get the right NPS prediction by measuring faux-ownership.
The scourge of generic courtesies
Generic niceties, such as, “I’d be happy to help you today.” pose the same risk for your AI measurement tools as Adam's delight to resolve. “Let me take care of that for you.” is one of the hundreds of generic courtesies I've compiled. These are the courteous, polite, personable, utterances of a friendly well-trained call centre team. It's not a bad thing at all, these are the basics, but this isn't ownership. And measuring generic helpfulness does not correlate with NPS. How do I know? I've done the math!
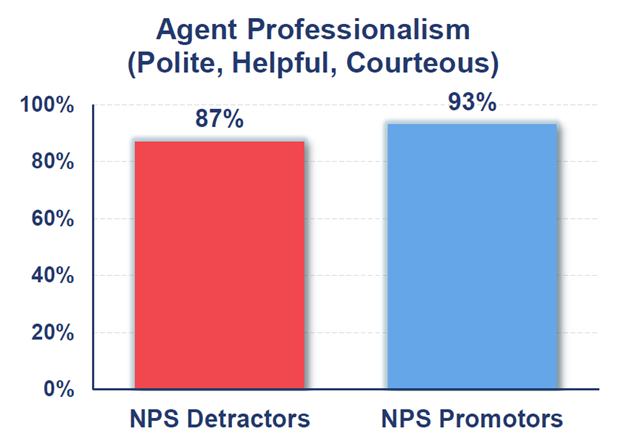
Doing the math
When looking at 10,000 Live Chat transcripts from Liveperson, there’s zero difference between Detractor and Promotor conversations in terms of agents being professional, friendly, helpful and courteous. And again, these are not the agent behaviours that make a difference. They are the basic hygiene factors necessary for working in a professional service organisation.
Just like canned empathy, they do not resolve customer frustration alone. Or QA-driven utterances like thanking the customer for their loyalty. Something that gets a near 100% hit rate in many USA service organisations on both promotor and detractor conversations. And your predictive NPS measures need to go beyond this.
Stop generic sentiment listening
Recall for a second, the above customer struggling to speak with the VIP team; she didn't say she was disappointed; upset, or frustrated. Yet we all can see she was super p*ssed with multiple contact attempts and the agent intransigence and unwillingness to help. There are hundreds and hundreds of sentiment-based tools. Beloved by marketeers and 'social listening' experts. But the sole purpose is to determine if the customer is happy or sad. Satisfied or dissatisfied, but they are not sufficient. If you run the transcript above through a sentiment tool, and it states the customer was neutral. One of the biggest problems in predicting NPS is that customers do not articulate dissatisfaction in the way you want to measure it.
customers do not articulate dissatisfaction in the way you want to measure it.
Again using 10,000 LivePerson Live Chat transcripts, the data below shows that using an out-of-the-box sentiment tool, dissatisfaction is picked up in just 10.4% of NPS detractor conversations.
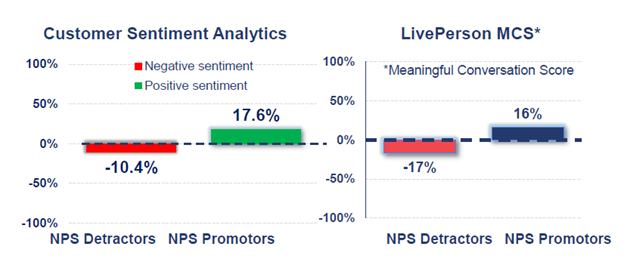
LivePerson has a built-in tool, the Meaningful Conversation Score (MCS). In the detractor sample, the MCS average score for the detractor population is -17%. Looking at sentiment just won’t get you the results you want. As the Director of Operations for a large UK bank told me, “Well, we look at MCS but we don’t take any notice of it.”
we look at MCS but we don’t take any notice of it
Based on the data I have, sentiment analytics gives you 17% of what's going on in a detractor conversation, and that’s just not enough. So, we've looked at risks of measuring canned empathy, trite agent application behaviours, faux-ownership and now, the risks of relying on sentiment analytics. So, let’s be a little more helpful, courteous, and polite and move on to what different elements should be considered to accurately predict NPS.
Predicting NPS using conversation science
Predicting NPS is hard; really hard. We started with 15 years’ worth of conversational data in our attempt to crack the challenge. We gleaned all agent utterances that were uniquely present in CSat1 (very dissatisfied) and NPS detractor calls and applied to a dataset of 10,374 LivePerson Live Chat transcripts.
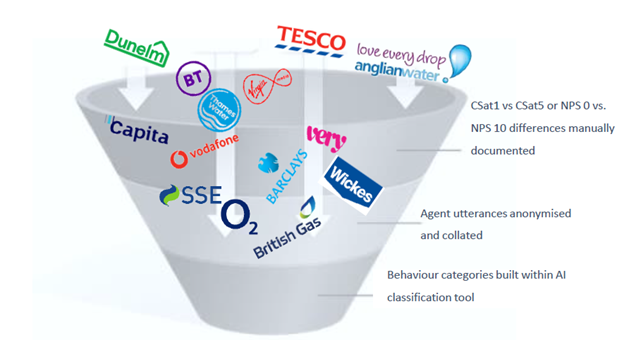
A model for predicting NPS
When we applied our model, we found three significant categories of measurable, discreet verbal ‘utterances’ within the LivePerson live chat data. Now, this isn’t about just the agent's behaviours. It’s a convergence of agent behaviours, implicit and explicit customer dissatisfaction and evidence of high effort for resolution pushed back to the customer.
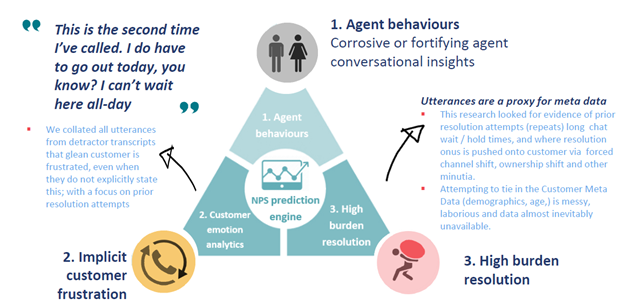
Multi-variate regression analysis (courtesy of the amazing Dr. Danica Damljanovic at Sentient Machines) shows these three elements make up 91% of the variability in the sample. We had to take an advanced statistical approach, otherwise, we wouldn't know what the hell was going on. As for the remaining 9% of variability, well, customers are irrational idiots; they don’t understand NPS surveys or how to use live chat! Also, they don’t like having a banking loan application turned down. A rejected customer accounts for 0.7% of NPS live Chat Detractors.
High Burden Resolution
Indeed, within live chat, the agent behaviour plays far less of a role than anticipated. Except in the area of intent resolution. Live Chat is truly a horrific channel for resolution and 42% NPS detractors had zero resolution and were pushed elsewhere. The Death of Live Chat goes into detail about what's going wrong here.
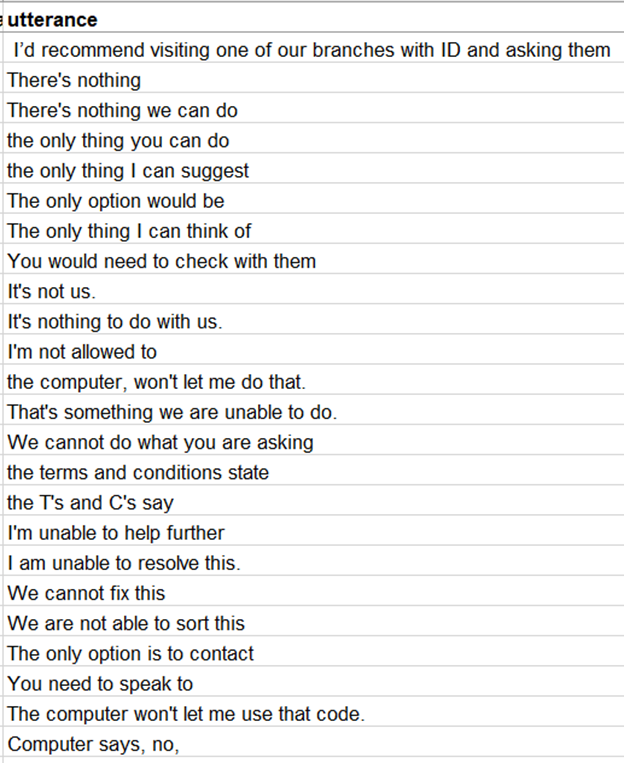
But here's an Easter Egg for you! You can mine your resolution failures easily by looking at the agent utterances. Above are some examples of what to look for. I can't share everything as it's 15 years' worth of intellectual property... but this is a good starter for ten!
Implicit Frustration
As we've explored, customers do not articulate dissatisfaction the way we want to measure it. But they do say things such as:
"I can't wait home all day."
"Nobody turned up with a delivery"
"This is the second time I've called"
"The webchat team said I had to phone."
So, the second element needed to map out are the common customer utterances that represent implicit frustration and evidence of repeat contact. Get your AI conversation designers on board, second (or commandeer) the QA team, and get some superstar team leaders involved. You'll soon have a list of 10,000 for each. And that's a damn good start for any enterprise operation. That's probably sufficient for 80% of all implicit customer frustration.
Mine your NPS verbatims; live chat detractor transcripts and anything else that's correlated with dissatisfaction. The initial agent conversations that generate an FCA registered complaint can be incredibly revealing too. In one £4bn company, 60% of all FCA complaints featured what we called agent intransigence. A "powerless to help" rally of, "there's nothing we can do."
And once you have your 80%; talk to your IT machine learning team about Paraphrastic Machine learning... this will get you to 99% painlessly by using some smart AI to find semantically similar phrases. You can buy this off the shelf too. There's only one company that I know of offering this as an after-market tool HumanFirst.ai
The plane crash agent conversations.
This is the final, and most neglected element of predicting NPS. But the most fascinating. In our research, there are four agent behaviours that only ever feature in NPS detractor conversations. These are the place crash agent behaviours. The behaviours that destroy the customer conversation.
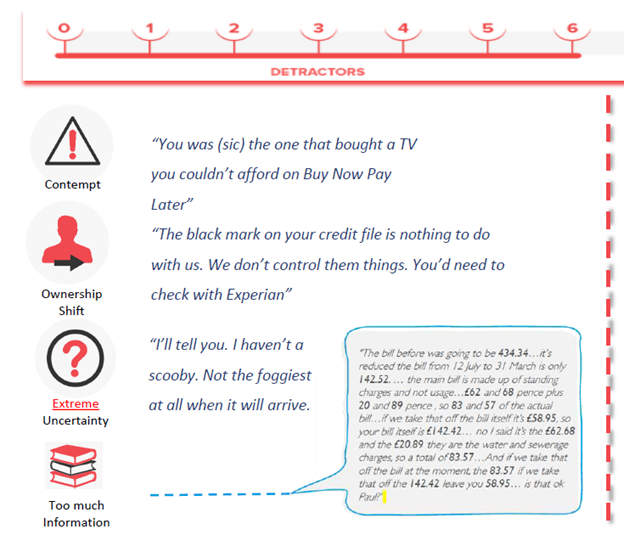
They are fundamentally self-explanatory. Although Too much information is a curious one. In an attempt to win over the customer with logic, well-intentioned billing teams can often overwhelm the customer with a deluge of financials. In the interest of brevity, I won't dive into the minutia here, but there's a separate long-read on these four behaviours
What positive agent behaviours should we measure?
Do you remember Adan from the BPO Web Chat team. It was Adam who was "delighted to resolve your query." But we know that's just faux-ownership and measuring this won't help us predict NPS. Let’s contrast Adam's trite opener with an absolutely incredible example of personal ownership from an agent at Tesco. They really have nailed agent ownership in their contact centre as a best practice, Let’s have a look at a real-world telephony example (I was the customer in this instance):
Customer: “Yeah. My, delivery driver didn't turn up. I waited here all morning. He was supposed to arrive by 11am but nobody showed up.”
Agent: “Oh, dear. That’s definitely not the experience we want to have. Don’t worry. I’m going to take care of this for you. What I'm going to do, if you wouldn’t mind just hanging on, is I'm going to phone the store. I'm gonna find out where he is and I will make sure the store calls you within 10 minutes to give you a precise update. Because otherwise, they will have me to deal with.”
Own what you can own.
This is ownership. Pure ownership at its finest. This wasn't a trite statement, “I would be happy to resolve this for you.” The agent gave her personal reassurance and projected absolute certainty.
Agents are often loath to make a personal commitment when they cannot own to resolution. The agent couldn’t physically deliver the home grocery shopping but she damn well made sure it happened.
The personal reassurance here is what sets the conversation apart from a trite, generic pre-programmed “ownership” dialogue and the “agent certainty” and precision around timescales is the secondary behaviour that correlates highly with NPS promotors.
Agent certainty
There’s also a really unique insight here that almost all enterprise firms miss when attempting to predict NPS or customer satisfaction. The requirement for agent certainty.
Have a read of this!
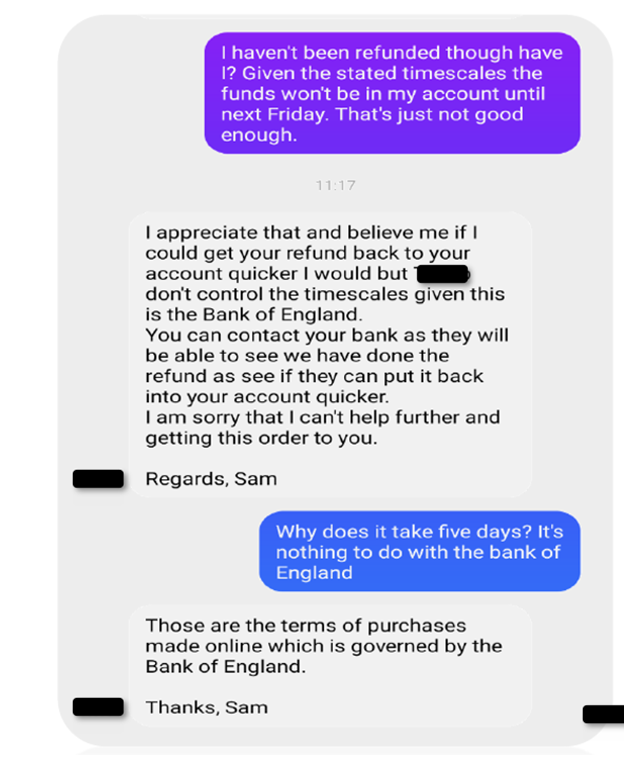
Your refund, of course, is not controlled by the Bank of England! We know that. But it’s a pretty messy explanation for most agents to give. I did meet one contact centre team, in William Hill contact centre that achieved a near 100% CSat rating in explaining why customer refunds took 3 to 5-working days. It was enlightening for me and this is the type of behaviour (or utterance) to measure with your speech analytics tool. In the interests of brevity, I won't share too much as I'm close to the 5,000-word limit already!
Moving towards a new era of conversation intelligence
The challenge today with predictive vendor solutions is not a tech problem. It’s a people problem. Well, more specifically, it’s a lack of understanding of human conversation science and a belief in the common wisdom around agent behaviours without actually ever-challenging these assumptions.
The worst combination I've seen is when the in-house L&D teams conspire with JavaScript developers to build and code the speech analytics measures. That's the kind of disaster that leads to rating 1,200 agents on how profusely they are apologising. Hopefully, you agree that canned empathy isn’t the solution. Sentiment Analytics doesn't work in isolation and customers do not verbalise dissatisfaction the way we want to measure it.
The good news is that most enterprise firms have a new breed of conversation specialists. Linguists that specialise in AI Conversation Design. Leverage that expertise to improve your speech analytics system. Corral your QA teams before they are made redundant by automation and re-purpose them for a much greater purpose!
I've tried to share a roadmap for what I believe to be one of the ways to predict NPS. Yes, it's a little laborious and not as sexy as using AI to glean the differences. But it will get you to the right destination. As ever, if you'd value an initial chat about all things conversation intelligence related, I'd love to speak. Those conversations never come with an invoice!
Survivorship Bias References
The Abraham Wald story has been severely dumbed down for the benefit of the internet and even TeD Talk audiences. The de-classified report is here. I don’t profess to understand it all. Pages 66, 74 and 88 are the most fascinating. A Method of Estimating Plane Vulnerability Based on Damage of Survivors by Arthur Wald 1943 (reprint 1980)
ABOUT THE AUTHOR
Andrew Moorhouse’s specialties include conversational intelligence; customer journey management; and AI speech analytics. A PhD candidate in conversation science, uses conversation science to “eke-out those difficult CX and revenue gains for human-and AI-call centre agents. His research and consulting expertise is the codification of human behaviour. He has worked on the most critical performance improvement projects for UK Enterprise clients such as Tesco, Vodafone, Anglian Water, Cisco, the VERY Group (Shop Direct), Thames Water, EE mobile (retail), Lex Autolease, Thales and O2-Telefonica.
END NOTES
1. See also definition of Net Promoter Score (NPS)