Written by Russ Edelman, Founder & CEO of Contracts 365,Inc. (formerly Corridor). Contracts 365 is a leading innovator in the Contract Lifecycle Management (CLM) industry.
Executive Summary
The impact of AI on Contract Management and the world at large has profoundly changed with the introduction of Large Language Models (LLMs) that drive Generative AI from companies such as OpenAI, Google and other industry heavyweights. And while all of these LLMs bring their own value proposition, ChatGPT by OpenAI has gained the most notoriety. The excitement is understandable, the skepticism palpable – and the practicality of bringing AI and Contract Management together is being assessed—and reassessed—because of GPT. This article looks at the opportunities that exist in integrating AI and Contract Management through the lens of practicality; a lens that often gets disregarded in the spirit of the hype, and the continued uncertainty surrounding GPT and LLMs. I hope when you complete the reading of this article, you’ll be much better positioned to appreciate the impact of AI and the role that it can play in your organization. You’ll also be able to discuss two key dimensions on the subject; AI Components, CLM Infrastructure and where the intersect through “Ten Target Areas” that allow for AI and CLM infrastructure to add practical value for your organization.
Consider AI prior to ChatGPT like a rowboat; it was largely a small, self-contained means of transportation. When ChatGPT hit, it introduced a new form of AI, one that transformed into a jet plane. While we all love to get places quickly and efficiently, the problem with a jet plane is that it needs infrastructure. It needs to be able to land, it needs to be serviced, it needs airports to facilitate people getting off and on in a predictable and manageable way. A rowboat doesn’t need any of that. But, without infrastructure, a jet plane will provide limited or no value at best—or result in a pile of flaming wreckage at worst.
Where AI serves as the plane, a Contract Lifecycle Management system would represent the airport and all other supporting processes and systems—the CLM infrastructure—that AI needs to add value to Contract Management. This article will explore these two distinct dimensions; a) the key capabilities associated with AI and b) CLM infrastructure. It will then explore where these two dimensions intersect; the “Ten Target Areas” defined below which allow AI and Contract Management to be optimally integrated together.
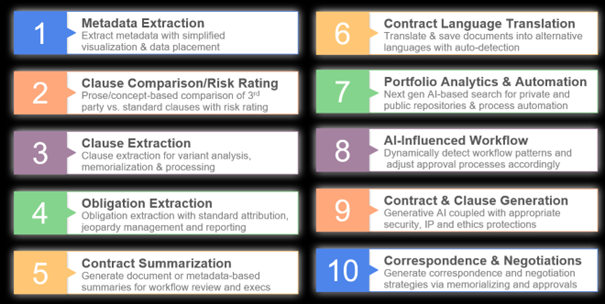
Key Takeaways:
• AI does not define Contract Management; it helps to transform it.
• There are two key dimensions to AI and Contract Management; AI capabilities, CLM infrastructure and at their intersection are the Ten Target Areas — make sure you have a clear grasp on them and how they interact.
• Having the proper security, IP and ethical protections in place is vital to the success of AI enabling CLM capabilities. Do not put all your faith in AI, at least yet. Employ a “Trust and Validate” mindset that allows you to confirm that the AI-infused portions of CLM are properly validated before accepting the AI outputs.
Setting the stage
Regardless of whether you think AI is good or bad, one thing is clear: it is here to stay. While AI has been kicking around since the 1950s, its incremental progress was limited, despite the massive investments and hype that it garnered throughout the years. Then OpenAI’s ChatGPT hit in November of 2022. And suddenly people began to incorporate AI into the fabric of how we live, learn, conduct business, and of course, contract.
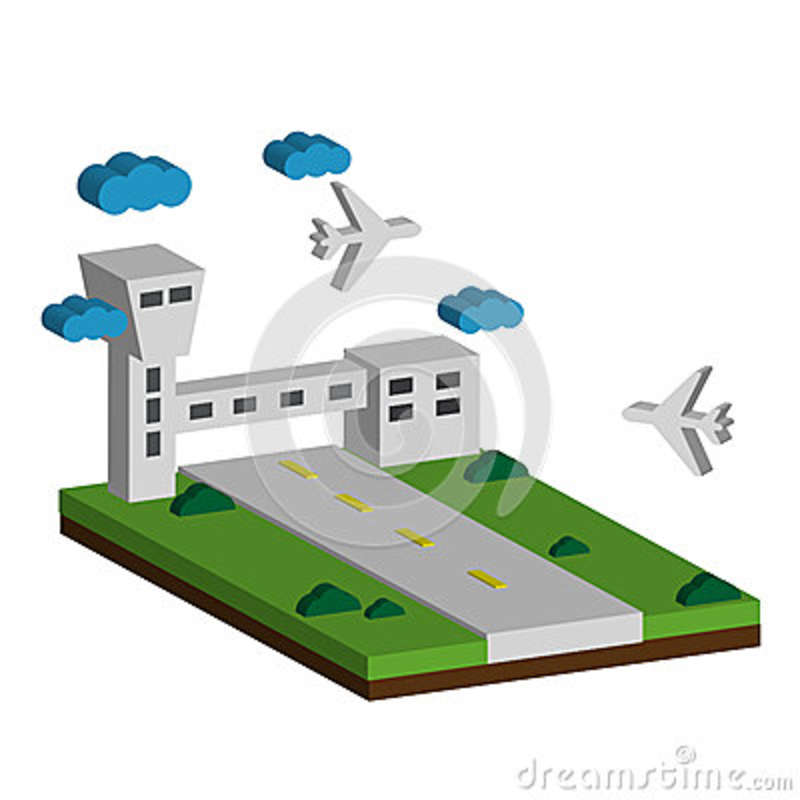
Zach Abramowitz, founder at Killer Whale Strategies, a legal industry consulting firm was recently interviewed on the Contract Heroes Podcast. During the interview, he commented on AI pre- and post-ChatGPT. He noted that, pre-ChatGPT, AI was like a boat moving along as fast as a boat can go. Post-ChatGPT, AI has been transformed into a jet plane and of course, the speed of a jet plane is far and away faster than any boat.
I think this is an apt metaphor, and one that can be built upon. For many applications, like CLM, AI is not just a jet plane—it is a jet plane without a landing strip, without air traffic control, the fuel and plane maintenance, without an airport, without proper airport security and safety procedures, without the air traffic controllers and so much more. Such a plane, one could easily surmise, will inevitably crash and burn.
So I’d like to use it to provide a meaningful context that sets the stage for organizations and contract professionals as they try to build a practical strategy for incorporating AI into their contract processing initiatives. First, we will explore the components of AI. Next, we’ll define the very necessary components of “CLM infrastructure”. Then, we’ll provide 10 optimal “target areas” where AI components would meet CLM infrastructure.
As another industry point on the impact of GPT, we now turn our observations to the increasingly high-profile debate on what percentage of attorneys will be displaced by AI. Casey Flaherty of LexFusion believes that 30% (or more) of attorney activities will be displaced in 3 years and Alex Hamilton of Radiant Law believes that 5% will be displaced in 5 years.
As a guy living in this CLM world for quite some time, I would most definitely fall into Casey’s camp of 30%. I think that number supports the notion of how the jet plane of AI is unquestionably real and getting faster in comparison to the AI boats of the past. I’d also add that the 30% impact is real in terms of traditional legal work. However, agile attorneys will see new opportunities to bring value to the table. Part of that formula will include building up skills in the use of AI, thus abating some of the suggested attrition for attorneys and effectively pivoting away from the mundane transaction processing to more high-value activities.
Within the realm of contract management, the successful and high-value use of AI in a business context has for the past 20 years been limited. CLM Software vendors who initiated their product strategy on AI are suddenly having to pivot due to the progress of Large Language Models (LLMs) like ChatGPT. Meanwhile, CLM vendors who initiated their product strategies with a more traditional CLM-Infrastructure approach (please see definition below) are now reexamining how to implant AI strategically into their existing products in light of GPT/LLM implications.
Practically speaking, the foundation provided by a CLM Infrastructure—i.e. the full lifecycle, data-centric, enterprise CLM—is still the primary requirement for enterprise contract management. An effective CLM solution facilitates the efficient flow of business-critical data around the organization providing real time information to those who need to know .
CLM solutions built with an "AI first" approach are likely to be missing critical elements required for contract management, and those vendors are buying time by showing the 'sizzle' until they can develop the ‘meat’ of their solution. On the flip side, if CLM Infrastructure vendors are not far along in their AI progression, they have the potential of being regarded as less progressive and not in-line with the interests of those buying and using Contract Management Software. It’s important to realize that, ultimately, a company can manage contracts at scale without AI; it cannot manage contracts at scale without CLM Infrastructure.
Please note that this article is not intended to be a deep dive into AI/ChatGPT/LLM capabilities. Rather, it is to shine a light on the importance of understanding how AI and Contract Management can practically co-exist. And after endlessly comparing notes, delivering AI-transformed contract management software and performing research with industry peers, the current and concluding theme is as follows:
Artificial Intelligence does not define Contract Management.
Artificial Intelligence can help transform Contract Management.
Practicalities of AI & Contract Management
The Plane | Artificial Intelligence Components
When deconstructing the various aspects of an AI engine, one can observe that there are several key components that serve at its heart as commented upon below:
- Input/Command Processor | Prior to ChatGPT, the techniques used for querying an AI platform were incredibly diverse with different interfaces; be it manual or programmatic. Then, along comes ChatGPT with a “Command Processor” as a new and easily adaptable interface for input/queries to get results from ChatGPT. Simply put, the world changed has changed with this simplified experience along with the output that comes from it and it will only continue to change more aggressively.
- Practicalities | The ChatGPT Command prompt is incredibly easy to use and delivers immediate results to the person issuing the commands. However, in order to maximize the output that comes from the commands, the user must be prepared to learn how to refine the commands that go into the command prompt. For example, your first command may be “Create a Non-Disclosure Agreement” and ChatGPT will deliver. However, you may want to extend the command to get more acute output. For example, “Create a mutual non-disclosure agreement for a life sciences company and a software company both located in Jurisdiction with a term of 2 years”. And this is simply a starting point. In the context of contracts, you may also need to take lengthy contracts and “chunk” them into smaller collections of content for ChatGPT to process. This becomes more challenging when provisions in certain “chunks” cross-reference provisions in other chunks and simplification of contracts must be considered as well. Allowances must be made to accommodate both chunking and simplification scenarios. With more sophisticated commands, more expertise is needed; however, the beauty of Command Processors is that it is not a programmatic effort, it is human based language. The complexities of large contracts (currently force chunking) or simplifying contracts for comparison purposes, may require developers to create an interface, which then creates the appropriate command prompts to consistently get the desired output. Also note that the actual amount of content you can enter at the command prompt continues to grow and ultimately, chunking may become a non-issue.
- Output/Results | When sitting at the command prompt, you will typically get natural language back when asking for ChatGPT to generate a contract or provision. In the NDA example above, ChatGPT will in fact generate a Non-Disclosure Agreement. Will it be perfect; most definitely not; however, will it be largely right with the understanding that some form of review and validation is needed; the answer is largely, yes. For purposes of other key contract activities such as metadata extraction, clause comparison, risk assessment and more, this is simply another example of a plane without an airport. The data may be successfully extracted; however, it becomes incredibly difficult and technical to then do something with it as the data is typically returned in a JSON (programming/structured) format and simply not consumable in raw form by non-technical people.
- Practicalities | As a general consumer/contract professional, be prepared to do something with the output such that it can be incorporated elegantly into your contract management software. As a developer/software company, be prepared to create a very elegant experience for your product.
- Security & IP | When using ChatGPT via OpenAI, the question of security and IP protection has appropriately become a focal point of concern. Whether OpenAI utilizes submitted command prompt content and ChatGPT produced output is hotly debated and it raises the specter of security and IP ownership. If for example, you load up two contracts for purposes of comparison, does OpenAI keep those contracts and use them for further training in their models? Who owns the IP for those documents? And will they circulate around even further outside of your organization?
- Practicalities | Of course, OpenAI has a security model. However, it should be noted that, by using the consumer tools (e.g. ChatGPT and DALL-E Labs) your input and output will be utilized to further improve OpenAI’s “model performance” unless the users explicitly opt out of sharing data. That said, if you are building applications that programmatically call the OpenAI API, your data is by default protected (OpenAI data usage policies link). Alternatively, OpenAI can be accessed via Microsoft Azure and Microsoft has taken extensive precautions regarding security and IP protection. Specifically, all of an organization’s content that is processed by Azure OpenAI does not leave that organization’s Azure instance (Microsoft Azure OpenAI Service data privacy link). This provides a substantially heightened level of protection and gives anyone using Azure the appropriate assurances in terms of security and IP protection. For the purposes of full disclosure, we (Contracts 365, Inc.) are a strategic Microsoft partner and leverage such services.
- Ethics | While the specter of poor ethics when using ChatGPT has been more heavily debated in academia, that same ethical conundrum of using ChatGPT for contracting exists. At a governmental, corporate and personal level, people are exploring the ethical conundrums of using ChatGPT. If for example, you use ChatGPT to generate a contract, to compare a contract, to perform a deep search for high-risk language, do you have an ethical responsibility to properly attribute the results of the output? And, if there is agreement in terms of ethical responsibilities, who and what is taking the appropriate steps to support proper attribution and/or constrain access to the results?
- Practicalities | To effectively support ethical boundaries, a complementary collection of technology and process must be adopted with a heightened emphasis on the process (as in teaching, learning, guiding). With technology, attribution can be more readily tracked; however, where it gets stored and how it gets automatically reviewed for compliance purposes needs to be factored into your use of ChatGPT.
AI is being used in many capacities and it’s certain that some of those uses will have applicability within the realm of Contract Management. It might lead you to ask, “Why is ChatGPT and the LLM construct different from last year and specifically the AI capabilities that were largely available prior to ChatGPT’s meteoric rise to prominence?” My response would refer to the boat versus jet plane metaphor. The power and sophistication of ChatGPT and other LLMs, despite their imperfections, is exponential orders of magnitude beyond their AI predecessors. And with all this power, you must be prepared to properly support it.
The Airport & Other Supporting Infrastructure | CLM Infrastructure
With the AI components referenced above, one must then ask, “Can’t I simply be a Command Prompt Engineer and drive my organization’s contract management initiative to untold heights with ChatGPT? And, of course, the answer would be, “No, stupid. Your plane will crash very quickly and so would your contract management initiative.” That’s because a sound CLM infrastructure must be in place to support the power of the AI engine. Turning once again to the plane metaphor, we need it to get people and cargo safely and expeditiously from one place to another. However, if the correct airport facilities are not in place, we have a problem. CLM Infrastructure, specifically in the context of AI, must support the following:
- Rich and Extensible Contract Metadata Construct | When AI has performed its work, the results/output need to be parked (stored) in a meaningful and manageable data storage location. This means that a flexible, secure and scalable data construct must be in place to store data. This includes extracted metadata, clause variants (when comparing third-party and standard clauses), obligations, AI-generated content and much more.
- Visualization, Validation, Transformation & Placement | It would be great if you could simply “turn on AI” and have it process your contracts. Unfortunately, it ain’t that easy. I fundamentally believe that ChatGPT/LLM is a game changer; however, you must employ a “Trust and Validate” approach—both now and for the foreseeable future. In our context, it means that when an AI process is run, such as metadata extraction, the data must be presented in a clean, elegant and very visually oriented way. This will help the person performing the process to better understand what they’re seeing. Jeetu Patel, EVP and GM, Security and Collaboration with Cisco states, “Don’t ignore UX in AI. It’s what creates the magic.” And I could not agree more – the visualization/UX is key. In terms of leveraging validated data, it means that the “UK” must first be transformed and validated into the United Kingdom. It means that the extracted data doesn’t simply get associated with one dimension of the data model/construct but rather, counterparty gets placed into a counterparty record, contract data gets placed into a contract record and so on.
- Processing & Notification Engine | Once the information is properly stored in the data construct, a powerful processing engine is needed to manage the workflow for approvals, signature, obligation tracking and escalation, risk management, automated alerting and much more.
- Analytics & Reporting | So many discussions around contract management start with the simple question of what type of reporting do you need to have in place to effectively manage your contract management process. This doubles down on the importance of the Data Construct, which serves as the accessible repository to enable your reporting. With a powerful reporting, dashboarding and analytics engine in place, data can be tracked, and workflow metrics can be applied, giving you the insights necessary to manage your contracting initiatives. It should also be noted that AI-infused search is becoming more important and will play a more substantive role in analytics and reporting. By harnessing AI to enhance search—to contextually find related relevant information that is not explicitly defined as part of a search criteria—is a key Target Area for AI and Contract Management Software systems.
- Robust Security | CLM infrastructure must include a powerful security model; one that supports Single Sign On, role-based permissions; with exhaustive security protections to ensure data and contracts are safe; and an extensive audit history appropriately preserved to ensure that all actions are fully memorialized. With the inclusion of AI, there is a need to provide a mechanism that allows for certain AI functions to be performed only by authorized people. For example, if contract generation via an embedded representation of the command prompt in the CLM system is made available, it needs to be constrained such that it is only available to contract professionals and/or attorneys. Additionally, once a contract or provision is created via embedded command prompt, the generated content must be elegantly captured, processed and secured. And the underlying exchange with the AI engine must comply with the appropriate data privacy protections that are provided by the AI platform.
Plane Interaction On The Ground | AI and CLM Integration Target Areas
The crescendo to our journey in this article comes from the actual landing of the plane in our airport with the safe and efficient unloading (and loading) of people and luggage along with the proper maintenance of the plane so it can live to fly again and repeat many more journeys. In practical terms, we have identified the ten most common “Target Areas” in which AI can help transform the contracting process; it lies at the intersection of the two dimensions, AI Components and CLM Infrastructure. Why identify these ten? Because a common set of definitions must exist for both providers and consumers of AI as we look to avoid the ambiguous question of “Do you support AI in your contract management software system?”. These are often referred to as post-processing activities.
These ten Targe Areas provide critical context that allows people to have a common dialog and understand how mature the AI capability is in the context of contract management. They also identify the value (high to low) of the use of AI in contract management. It is important to note that without the CLM infrastructure, AI will simply fall short of delivering meaningful and measurable value.
Target Area
|
Defined
|
Maturity
|
Value
|
Automated
Metadata Extraction
|
Ability to extract key metadata from contracts using little to no model training and to address the visualization, validation, transformation and placement of the extracted data.
|
High
|
High
|
Clause Comparison & Risk Rating
|
Provide automated 1) clause categorization followed by 2) comparative prose-based clause comparison against “standard” clauses with 3) ability to rate the complexity/associated risk of the counterparty-suggested language.
|
Moderate
|
High
|
Clause Extraction
|
Extract clause variants that meet pre-defined criteria and place into Data Construct with support for clause specific approvals, reporting, analytics, alerts and escalations.
|
Moderate
|
Moderate
|
Obligation Extraction
|
Extract obligations into the Data Construct with key data attributes being memorialized automatically via AI-processing. This is then available for automated obligation tracking, jeopardy management and reporting.
|
Moderate
|
High
|
Contract Summarization
|
Ability to auto generate a contract summary whereby the summarization can be stored as a rich metadata text field or as a summary document. This can then be incorporated into a workflow process, typically for users and executives to understand key points in a contract.
|
High
|
Moderate
|
Contract Language Translation
|
Workflow or on-demand based translation of a contract from one language to another. Note that the confidence level associated with the translation is not to be fully trusted as local colloquialisms need to be taken into account and, in this capacity, it is suggested that a validation process is introduced to ensure that the integrity of the translated contract is intact.
|
High
|
Moderate
|
Portfolio Analytics & Automation
|
This is a two-step feature. The first step is to provide a next-generation search experience that finds relevant contract information using AI-processing in your “Private” data repository as compared to the publicly available information on the internet. The second step feeds the results into an automated action/workflow. For example, step 1 search analytics may produce an inventory of contracts out of compliance and step 2 links the search results into automated amendments with e-signature integration.
|
Low
|
High
|
AI-Influenced Workflow
|
AI is used to observe workflow patterns and passively or actively adjust workflow to accommodate the pre-defined patterns. For example, if a common workflow pattern for standard contracts using your paper does not need a legal review, the AI-influenced workflow may a) suppress the legal review task and b) memorialize the bypassing of the legal review task in the audit history based upon X number of other instances not needing the same review.
|
Low
|
Moderate
|
On-Demand Contract and Clause Generation
|
Contract Pro-specific capability which allows for someone to initiate the creation of a draft contract or clause via ChatGPT and, once approved, converts to a modular and standardized template or clause. The premise of this Target Area is that the On-Demand portion is delivered by exposing an in-context command prompt to GPT. The Contract Pro could then issue command prompts with refinement and when the correct content is produced, it should be saved into a secured area in the Data Construct which can then provide for additional review cycles via workflow.
|
Low
|
Moderate
|
On-Demand Correspondence & Negotiation
|
Contract Pro can use AI to automatically create standardized and contextual correspondence during contract negotiations. As part of this process, the negotiation correspondence is captured into the Data Construct and this may then initiate approval and review cycles.
|
Low
|
Moderate
|
As we wrap up this article, my hope is that it provides you with a framework that can legitimately be used to assess your AI Target Areas and to ensure that both the AI components are available as well as the CLM infrastructure needed to support your AI-powered contract management software. As you go down the road of assessing what is best for your organization, please note the following four key takeaways:
- Check for CLM Infrastructure | Using AI to help transform your contract management process has become achievable with more impactful results. Make sure the CLM infrastructure is properly in place to accommodate your use of AI.
- Target Area Identification | Identify Target Areas that are of most value to your organization. For example, if the majority of contracts you process are on your paper, the value of Metadata Extraction will be substantially diminished.
- Trust & Validation Mindset | If you believe this will all magically happen without someone validating the output of AI, you will be disappointed; at least for now. Make sure you put in the proper validation processes and look for CLM systems that provide for Visualization, Validation, Transformation and Placement.
- Do Not Over Promise | While the reality of AI transforming Contract Management is finally here, please do not overpromise capabilities and assume there will be no involvement from your people. Attorneys, Contract Professionals and people engaged in the contract process need to learn new skills, deal with inaccuracies and learn how to handle these in efficient ways. AI-powered Contract Management is an entirely new way of working that will require proper change management and a necessary paradigm culture shift.